Designing your fMRI experiment¶
A full treatment of experimental design for fMRI research is beyond the scope of this handbook, but we try to provide a high-level summary with pointers to other resources; e.g. cite:huettel2014functional. Make sure to plan out your analyses prior to collecting data, as your planned analyses will ultimately constrain your design.
Most fMRI experiments fall into one of three categories: (1) block or event-related tasks, (2) resting-state, or (3) naturalistic paradigms. You probably already have a general idea which kind of paradigm(s) you need, and many projects use multiple paradigms (e.g. collecting both task and resting-state runs). Each of these categories has some special considerations to keep in mind.
Resting-state¶
In resting-state experiments, participants are not presented with any particular stimuli and are simply asked to keep still. Given the absence of an external stimulus or task, resting-state data are typically analyzed according to the covariation of intrinsic fluctuations among groups of voxels—i.e. functional connectivity and network analysis. The functional connectivity analyses used with resting-state data are especially sensitive to head motion and physiological noise, and therefore rely on more stringent noise mitigation; e.g. confound regression, scrubbing [Power et al., 2014] [Ciric et al., 2017].
Special considerations for resting-state:
What is the duration of your resting-state scans? Note that longer scans (e.g. 10 minutes) yield more reliable connectivity estimates [Birn et al., 2013].
What are your explicit “task” instructions? For example, will participants be instructed to keep their eyes open and maintain fixation? [Patriat et al., 2013].
Will you use online motion monitoring (e.g. FIRMM) during acquisition?
Naturalistic paradigms¶
Rather than using tightly controlled task designs, some researchers use more naturalistic stimuli (e.g. movies, stories) in hopes of increasing the ecological validity of their findings. Naturalistic stimuli are difficult to exhaustively model and any given variable of interest is likely correlated with other variables. While traditional task data are typically designed to test a particular hypothesis, naturalistic data are typically rich enough to inform a variety of hypotheses.
Special considerations for naturalistic paradigms:
Many naturalistic stimuli (e.g. movies) are quite long. How are you going to break up the stimuli? For example, you may wish to divide a 2-hour movie into eight ~15 minute segments.
Naturalistic paradigms typically have lower statistical power to detect a specific effect than controlled experiments designed to isolate that effect [Hamilton & Huth, 2020]. Nonetheless, you should pick a naturalistic stimulus that includes variation along your variable(s) of interest.
References and resources¶
- Aguirre, 2007
Aguirre, G. K. (2007). Continuous carry-over designs for fmri. Neuroimage, 35(4), 1480–1494.
- Aguirre et al., 2011
Aguirre, G. K., Mattar, M. G., & Magis-Weinberg, L. (2011). De bruijn cycles for neural decoding. NeuroImage, 56(3), 1293–1300.
- Birn et al., 2013
Birn, R. M., Molloy, E. K., Patriat, R., Parker, T., Meier, T. B., Kirk, G. R., … Prabhakaran, V. (2013). The effect of scan length on the reliability of resting-state fmri connectivity estimates. Neuroimage, 83, 550–558.
- Ciric et al., 2017
Ciric, R., Wolf, D. H., Power, J. D., Roalf, D. R., Baum, G. L., Ruparel, K., … others. (2017). Benchmarking of participant-level confound regression strategies for the control of motion artifact in studies of functional connectivity. Neuroimage, 154, 174–187.
- Hamilton & Huth, 2020
Hamilton, L. S., & Huth, A. G. (2020). The revolution will not be controlled: natural stimuli in speech neuroscience. Language, Cognition and Neuroscience, 35(5), 573–582.
- Kriegeskorte et al., 2008
Kriegeskorte, N., Mur, M., & Bandettini, P. A. (2008). Representational similarity analysis—connecting the branches of systems neuroscience. Frontiers in systems neuroscience, 2, 4.
- Patriat et al., 2013
Patriat, R., Molloy, E. K., Meier, T. B., Kirk, G. R., Nair, V. A., Meyerand, M. E., … Birn, R. M. (2013). The effect of resting condition on resting-state fmri reliability and consistency: a comparison between resting with eyes open, closed, and fixated. Neuroimage, 78, 463–473.
- Power et al., 2014
Power, J. D., Mitra, A., Laumann, T. O., Snyder, A. Z., Schlaggar, B. L., & Petersen, S. E. (2014). Methods to detect, characterize, and remove motion artifact in resting state fmri. Neuroimage, 84, 320–341.
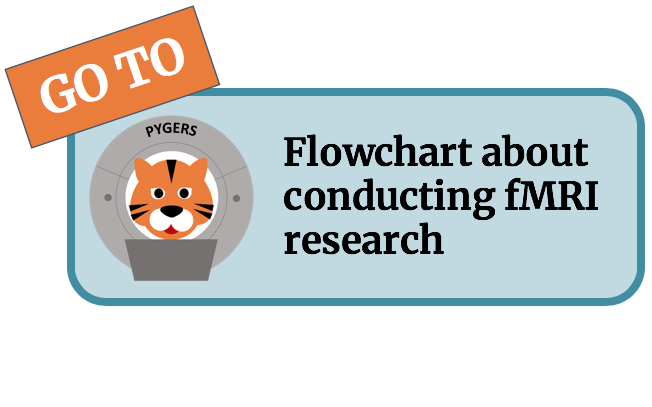